Terrorist pixel attacks of the future
2022/09/01 López-Gazpio, Iñigo - Adimen artifizialeko ikertzaileaDeustuko Unibertsitatea. Iturria: Elhuyar aldizkaria
With each moon full artificial intelligence becomes an increasingly important part of human beings. There is no doubt that artificial intelligence has conquered in recent years implausible goals, while these intelligent systems have some dark aspects that do not stimulate interest in becoming public in society. In this article, I'm going to talk about a weakness of image processing systems, pixel attacks, to get to know a less positive aspect of neurally-based intelligent systems and understand the risks of these image processing systems. Surely after reading this article you will not want to go back to sleep in your autonomous cars.
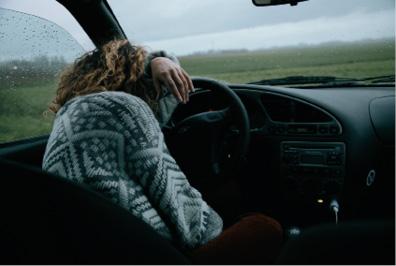
Basically, pixel aggression is about reversing the regular training of neural networks. In normal situations, to train a neural network system, you use a huge range of images that teach the system and learn to interpret what's in the image. In this operation process, the system parameters are adjusted with the wrong predictions of the neural network, which allow continuous improvement of the system results during the training period.
Although they initially make a lot of mistakes, after they've been learning enough time, the systems get a lot better until they're completely adjusted. Currently, image recognition systems offer, in general, very good results; in many cases, they are able to recognize almost 100% of images if there are no foreign objects between them [1]. When the neural network can't adjust anymore, the training is interrupted, the system is evaluated, and production takes place. These intelligent systems, fully fitted for cars, are part of autonomous car navigation systems, among others, to set up the car in a way that enables vision. The figure below shows an image of a recognition system for an autonomous car. As you see in the image, these systems allow the car to identify objects, people, animals, etc. It's something that's around you.
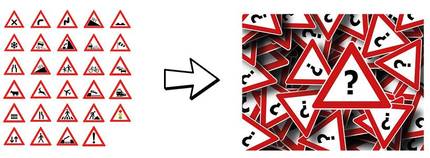
Using all this information in an introductory way, self-employed cars decide to make navigation in the best possible way and with the greatest possible safety: if there are risks, slow down or stop speed, speed up if the road is free and speed limits are not exceeded, move if the right lane is free, slow down and displace cyclists to advance them, etc.
On the contrary, if the adjustment of the neural network is turned upside down…
There's a possibility of fooling neural networks by modifying the input images. That is, if you revert the training process of the neural network by showing the network a false image and a target, you can get the disruption that you have to do in the original image to make you believe that you've deceived the network and that you've actually shown another image. These kinds of measures that can be made to artificial intelligence are very dangerous, for example, because safety signals to slow down speed or stop it can become signs of acceleration. The following figure shows a simple example of pixel attack. As you can see, if you add noise to a traffic ban signal, it becomes one more signal for an intelligent object recognition system, although the human being cannot see this change with the naked eye.
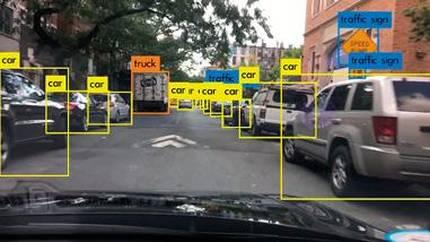
This phenomenon is called an attack on the opposite image and constitutes a significant line of research for those who are currently investigating the safety of the autonomous car. This imaging technique and systems fraud opens an interesting line of research on the trust and evaluation of intelligent systems.
So it can be interesting to investigate the amount of disruptions that need to be added to the images to trick a smart system. Samsung's research study by Kevin and his collaborators is the most terrifying of all written research on this subject. This article underlines that the level of disruption to be added to deceive a neural network is very low [3], which is a serious problem for future users of autonomous cars. In this research work, the authors show that a small change in a sign of use such as graffiti is enough to set up an autonomous car system that functions as a genuine fraud. It is enough to use a white and black ribbon when converting a stop sign into a limitation signal. It's terrible. The following figure outlines the specific case to which the authors refer in the article.
What can be done to protect neural network systems from these kinds of attacks?
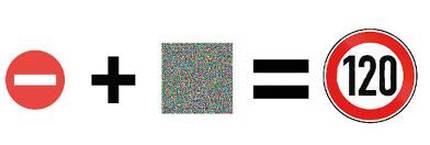
Recent research has shown that adverse image-based attacks do not depend on neural network systems, but on data sets used to adjust them. That is, they are a characteristic of the data set. This means that the adapted samples that serve to trick an architecture of neural networks are valid to trick another architecture, provided they have shared the same set of adjustment data on the date. Since the generation of large data sets is an expensive and complex process, it is very common for many neural network systems to be adjusted to the same data sets. This implies that the influence of the technique of the opposite image can be very serious and that the forms of protection of this type of image must be studied.
Counter-learning is the most well-known method of protection. It's pretty simple, although we don't have all the certainty that it supports us. With this technique a robust and anti-fraud neural network is built, the data set is completed with numerous examples of opposition. This allows the model to abandon the fragile or weak features of the process and learn to rely on stronger traits to make predictions. The success of this technique requires the massive creation of perverse and opposing examples. But the difference is that at the moment of a neural network, you can slow down the phase from three to 30 times, because the dataset massively increases with these kinds of images.
Researchers currently have tools to complete data sets with malicious images such as FoolBox. With this tool, you can generate malicious images automatically, and our intelligent system can be aware of the existence of this kind of malicious. However, it seems that this is becoming a war between aggressors and defenders, each of whom designs a newer technology to dominate the other.
Is this all the fault of artificial intelligence?
As we've seen, the technique of adverse imaging can produce very serious problems in situations where safety is so important, and the last neural networks are blowing. This is because neural networks are based on weak characteristics and don't understand or study image well. But the same problem happens to humans, because our useless brain does similar tricks to us when we attack images like optical illusion.
If you look at these kinds of optical illusions, at first it seems that the lines are not parallel, but when you look at them closely, these lines are parallel to each other. Like us, neural network systems also need this point of attention to be aware of the tricks that opposing figures want to impose. In fact, opposing images and pixel attacks are just images that force us to see things that don't really exist.
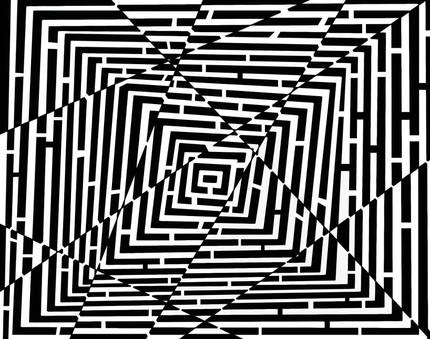
In the coming years, the development of new marketing and marketing systems will be the continuous game of the cat and the mouse. This will ultimately lead to more robust and reliable models, constituting an important step towards critical safety applications such as autonomous cars. For the time being, however, it is better not to get too far from the hands of the steering wheel in case.
References
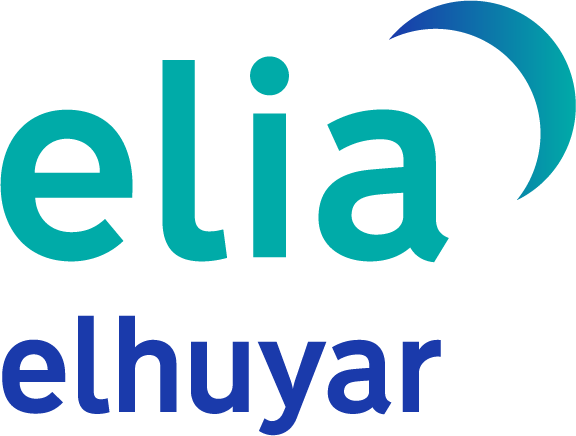
Gai honi buruzko eduki gehiago
Elhuyarrek garatutako teknologia