They propose a metric to quantify the demographic biases of the AA data sets
2024/03/05 Galarraga Aiestaran, Ana - Elhuyar Zientzia Iturria: Elhuyar aldizkaria
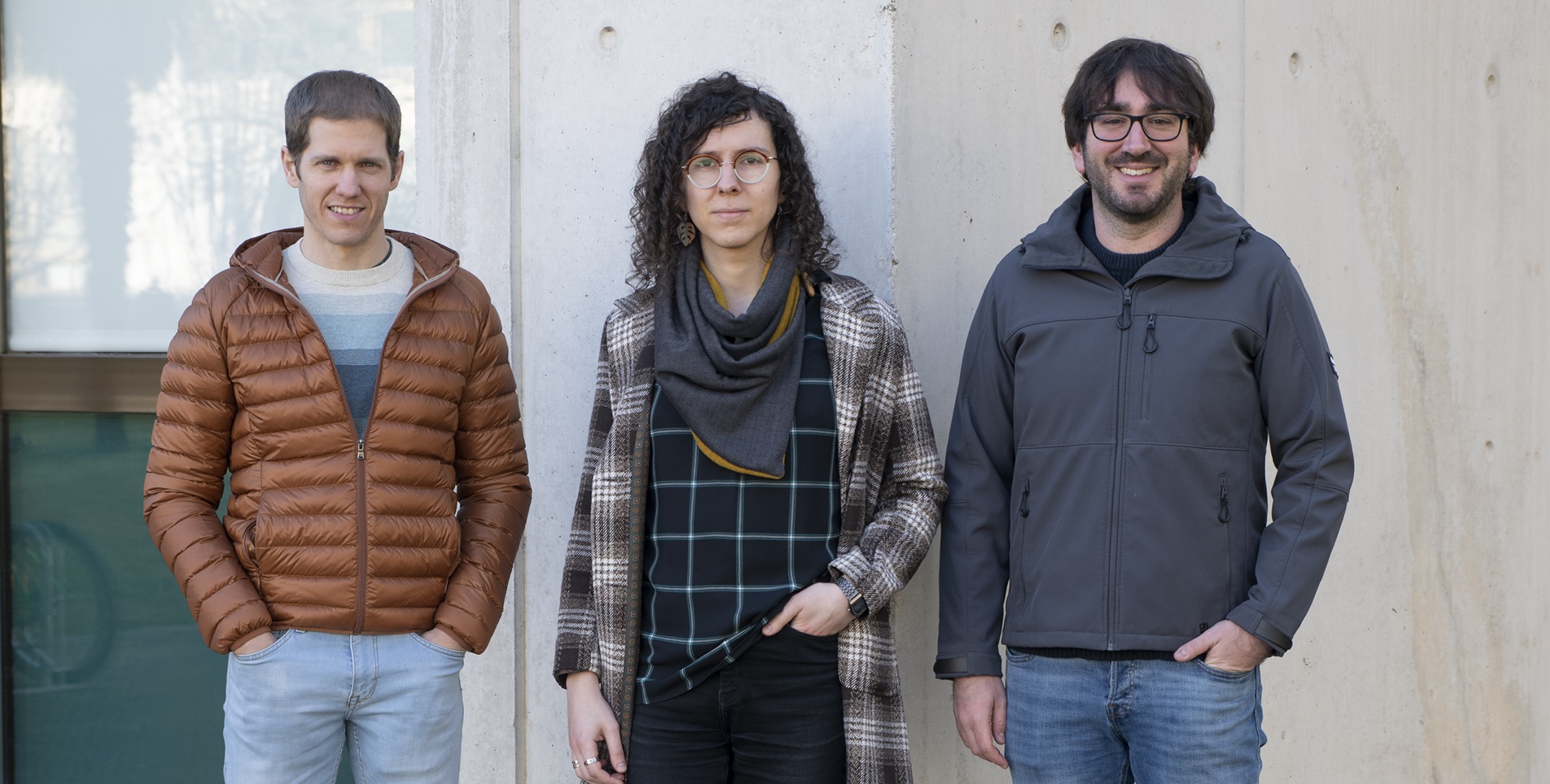
Informatics and members of the ISC Smart Cities Iris Domínguez – Catena Institute (NUP) have designed metrics to quantify demographic biases of data sets used for training artificial intelligence models.
And that is that, as you have explained, artificial intelligence systems have few or misrepresented certain demographic groups, such as women, people over 70 and black people. This may cause artificial intelligence systems formed with these data to act incorrectly and be treated discriminately by certain population groups.
For example, researchers have stated that some curricular filtering systems systematically excluded curricula that seemed female. And in the case of generative artificial intelligence, like the ChatGPT system, you've realized that it associates gender to certain professions, while some racial groups associate it to certain negative characteristics.
The research, published in the journal IEEE Transactions on Pattern Analysis and Machine Intelligence, is based on the automatic knowledge of facial expressions, that is, of the systems that invent in people's photographs what emotions they represent. These systems have important applications in medicine (detection of painful signals in infants), support robotics (especially for the elderly) and audiovisual creation.
More than one kind of bias
Thus, more than twenty data sets used for training the aforementioned systems have been analyzed. And you've seen that in the datasets, the presence of men and women is usually balanced, but not age and race. In fact, data from white people between the ages of 20 and 30 are much more than from other groups, and consequently, artificial intelligence models can discriminate against people over 70 and rationalized women, among others. These biases are called representation biases.
But it's not the only bias that artificial intelligence systems have. In fact, it is found that the number of happy women in many data groups almost doubles that of men, while the number of angry women almost half. This suggests to the system that a person's gender or sex is related to happiness or anger. They're called stereotypical biases.
In total, 17 metrics have been analyzed to quantify all types of bias, from which we have deduced which are the most adequate to quantitatively measure the biases contained in a data set, a first step to avoid the transfer of bias to artificial intelligence models and minimize its impact.
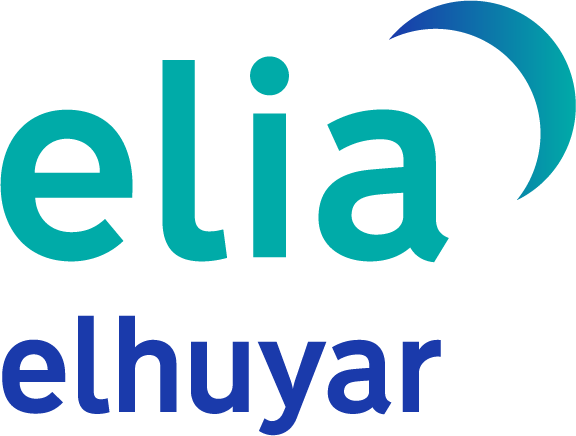
Gai honi buruzko eduki gehiago
Elhuyarrek garatutako teknologia